The EPA estimates between 6 and 10 million lead service lines exist in the United States. Despite the prevalence of lead pipes across the country, many towns and cities lack accurate and consistent records on service line material. Physical inspection through digging is the most reliable way to determine if a service line is lead, but inspections can be costly, ranging between $500 - $2,000 or more per property. Many communities are turning to digital methods such as using Artificial Intelligence to streamline executing these critical programs. But of course, as with any new type of technology, we need to understand its value.
Here are a few of the most common questions about using Artificial Intelligence to identify service line material.
Frequently asked questions
Why use Artificial Intelligence for predicting the probability of lead?
Artificial Intelligence (AI) can be an effective tool in predicting the probability that a service line is lead, making expensive physical inspections more targeted and cost effective to avoid unnecessary digging. The cost saving potential is compelling - for a city with 10,000 properties to inspect, a model that saves 10% of total inspections by providing reliable service line material predictions would lead to savings of $500,000 to $2,000,000 or more, depending on inspection costs per property. Leveraging AI to predict service line materials can improve the hit rate of inspections, optimize limited funding, and support effective prioritization and planning of inspection and replacement projects. For example, the cost to mobilize and demobilize field crews for inspection and replacement of lead lines can be optimized with appropriate planning.
.png)
In addition to predicting the probability of lead at the individual property level, AI models can be used to generate the probability of lead pipes at the street and block level. AI can also be used to predict the total count of lead pipes for a service area or by neighborhood, which is critical to understand how much funding will be needed for lead service line replacement. These types of insights can be visualized spatially, so that areas of probable high lead density can be prioritized.
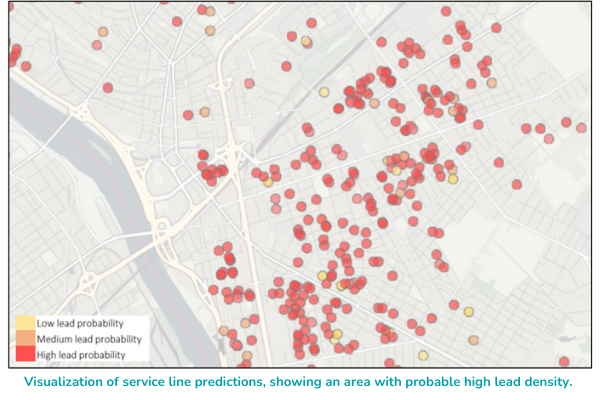
What’s the approach to using AI models to predict service line material?
Applying AI models to predict service line material is a phased approach. It typically starts with a representative sample of verified pipes from which the AI model can learn about patterns associated with service line material (the training data set). It can be costly to verify a subset of pipes for an initial representative sample, so in some cases, historical records, if considered accurate and up-to-date, can be used to train the model. This initial training data set is used to create a baseline model (the first phase). In subsequent phases, the model is improved with more field-verified data as the verification or lead service line replacement program progresses.
>>YOU MIGHT ALSO LIKE: Elements to digitizing the Newark Lead Service Line Replacement Program
How many sample records are needed to get started?
Determining the number of sample records needed will certainly vary on a case-by-case basis. For initial planning and prioritization exercises, it’s possible to get started with enough samples to achieve a 95% confidence level. The Michigan Department of Environment, Great Lakes, and Energy provides a table with the number of samples required to achieve 95% confidence level by system size in Appendix A of this guidance document.
To achieve reasonably high prediction accuracy needed to label services as non-lead, more samples may be needed. This will vary by community, but in general we would like to see at least 10-20% of the system verified to achieve reasonably high prediction accuracy (80% or greater). In some cases, however, it’s possible to achieve high accuracy with less than 10% of the system verified. For example, Trinnex and CDM Smith staff built a model built to predict service line material for a city in the northeastern U.S., where an initial sample size of about 200 verified pipes (less than 10% of the system) was needed to create a baseline model with >85% accuracy. The baseline accuracy increased as the program progressed and more labeled data became available to support the model’s learning.
The number of sample records needed will vary from city to city, and it heavily depends on the quality and other characteristics of the data that is feeding the AI model. In some cases, trends and patterns in the data are easier for the AI model to detect and learn from, which means a smaller initial sample size will be needed to produce reasonable predictions. In other cases, the opposite will be true, and more data will be needed to produce a reasonable baseline model.
What other data sources can help determine pipe material?
Data provided by the utility can be supplemented with publicly available data and 3rd party data, to build robust models for predicting pipe material. Data from the following sources helps to gain as much information as possible about pipe materials and the patterns and trends associated with pipe materials.
- GIS database
- CMMS (maintenance) database
- Paper records
- Assessors database and/or 3rd party housing data
- Data from meter replacement programs and water main replacement programs
- Interviews with retired operators, to obtain institutional knowledge
- Historic municipal plumbing codes
- Demographic data
After collecting available data, data pre-processing occurs for optimal modeling. This includes performing quality control checks, inspecting the data for unusual or unexpected values, and assessing the completeness of the data. As a critical step, AI experts also perform analysis to understand the critical factors in identifying the presence of lead pipes, using statistical methods as well as visual data exploration.
>>RELEVANT BLOG: 3 Tips for Delivering Intuitive and Visually Compelling Analytics
How do you ensure the performance and accuracy of an AI model?
With so many data inputs in play, it’s important to “cut out the fat” in an AI model to not only remove duplicate data but also align data that might be similar in nature. To support this, feature selection (reducing the number of input variables to help improve model performance) and feature engineering (creating new, more simplified variables to help speed up and enhance model accuracy) are critical steps.
Different methods are used depending on the type of variable (categoric or numerical). Duplicative explanatory variables (i.e., attributes that explain the presence of lead but are correlated with one another) are removed, otherwise they tend to cause unexpected and unreliable results. Depending on the data set, variables might also be combined, or their values transformed or consolidated into categories to support the model in more optimal learning. This is both an art and a science that requires the right level of experience. Over-engineering can cause unintentional information loss, but under-engineering can lead to sub-optimal model performance.
How do you select the best AI model for pipe material prediction?
Selecting the best AI model for pipe material predictions is an intriguing element of data science. The process starts by evaluating performance and reliability of up to 15 different types of AI models before selecting the best fit for the data set at hand. While certain trends tend to pop up in the types of models that seem to work best in predicting service line material, there is generally enough variation in the format, quality, size, and shape of the input data to merit a case-by-by case approach.
Like most technology solutions, applying AI to predict service line material is not a silver bullet. The effectiveness and reliability of the predictive model is dependent on the quality and consistency of the input data (the data used to generate predictions). Depending on these factors, different kinds of data pre-processing techniques can be deployed to enrich data that is used to train the AI model. Also, there is no one-size -fits-all solution. Although the training data sets will share some similarities from city to city, they are different enough to merit case by case evaluation and solutioning. Even if many cities collect the same kinds of data about their service lines, differences in history and regulations from city to city can create meaningful variation in the data. What worked for one data set is not guaranteed to work for another.
What if I don’t have in-house resources to create or manage AI models?
Leverage AI experts who’ve done the work (specifically with service line material predictions). Trinnex and the leadCAST platform were created specifically to help utilities manage their lead service line programs, from identification of materials to managing replacement program progress. The Trinnex team has experience applying AI models to solve a myriad of complex infrastructure challenges. Reach out today to learn more.
P.S. Need help with complying with the Lead and Copper Rule Revisions or managing your Lead Service Line Replacement Program? Our parent company, CDM Smith, has done plenty of work there, including serving as the program manager for the industry-leading Newark Lead Service Line Replacement Program.
Sources:
https://www.epa.gov/ground-water-and-drinking-water/lead-service-line-replacement