What is Random vs. Representative Sampling?
A “randomly selected” sample is not the same as a “representative” sample. “Random sampling” refers to a method where every member of the population has an equal chance of being selected, but this can sometimes lead to a lack of representation from certain subgroups (strata) within the population. “Representative sampling” is a method where the sample is selected to reflect the characteristics of the larger population, ensuring that all subgroups (strata) are proportionally represented.
“Randomness doesn’t guarantee representativeness.” To illustrate this concept, the figures below show an empirical analysis that our data scientists created with data from a community we are working with in the northeastern United States, to predict service line material and find lead service lines more efficiently. This water system did not have any existing field verifications to support predictive modeling, so we recommended an initial list of properties for them to field verify the service line material.
We experimented with two techniques; a simple random sampling technique and a representative sampling technique (such as the technique we use in our leadCAST Predict Inspection Optimizer tool). For the latter, we selected a verification set that is representative of the unknown service line materials with respect to property age and census tract. This results in many groupings of properties (for example, homes built during a certain time period, in a certain census tract). The bar chart below shows the proportion of properties in the random vs. representative verification set compared to the “population” of unknowns for a handful of these groupings.
This illustrates two things: 1.) With the representative verification set, the proportion of each group is much closer to that of the population of unknowns (the purple bars are much closer to the teal bars than the orange bars are) and 2.) Group 4 is not represented at all in the random verification set, which creates a clear bias.
In this case, “Group 4” is a group of properties within a certain census tract that were built during a certain time period. Imagine if it was an area with different development history from the surrounding areas, or a small water system that was acquired by the utility many years ago. Either way, the fact that it is not present in the random sample is a problem. A model built using the simple random sample in this example would be biased because it could not be used reliably across all groups within the population of unknown services.
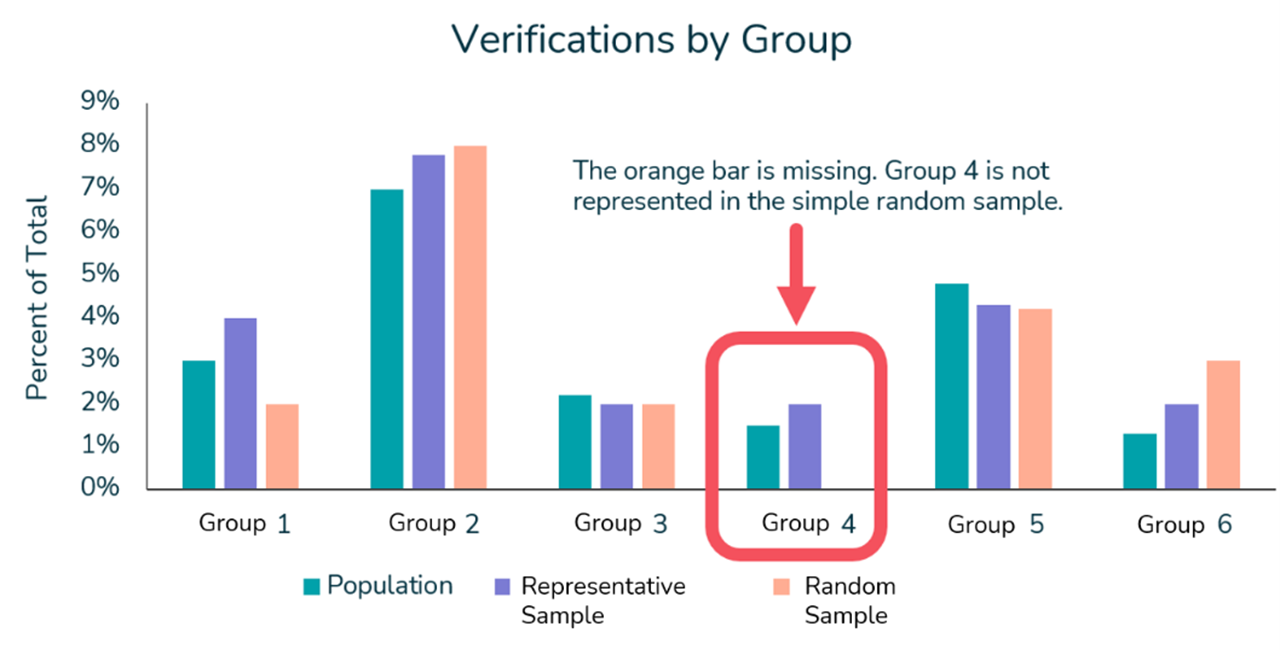

What this Means for Your Utility
If you are a utility who is actively seeking to better understand your service line inventory, predictive modeling using a representative sample helps you to make more reliable and unbiased estimates, target field verifications, and find lead service lines more efficiently. This ultimately saves time, money and leads to more streamlined protection of public health.
Trinnex® provides a representative field verification set collected for reliable predictive modeling, via leadCAST® Predict’s Inspection Optimizer. The tool helps us identify some of the top variables that are associated with service line material, which are unique for each water system that we support. We use this information to generate an initial set of properties for field verification, which are representative of the unknowns in the water system with respect to things like property age and value as well as population demographics.
Interested in achieving digital resiliency at your utility? Contact a Trinnex Expert today!