Trinnex hosted a webinar on June, 2022 to help utilities better understand how machine learning can help accelerate Lead & Copper Rule Revisions (LCRR) compliance in 2024.
The hour-long webinar covered topics including:
- An overview of the LCRR timeline
- A breakdown of service line inventory requirements
- A deep dive into the inventory development workflow including desktop studies, field investigations, and machine learning
>> Watch the full webinar here
Overview of the LCRR timeline
Greg Brazeau, VP of Sales at Trinnex, starts the webinar by providing an overview of the LCRR compliance timeline. Most utilities are currently in the materials inventory data-gathering phase. Greg points out that if lead exists, utilities also must prepare a lead service line replacement plan as well as public education and outreach programs:
- By October 2024, you must start notifying ratepayers about service lines with the “unknown material” status or with confirmed lead
- By January 2025, you must begin compliance and school sampling
- By July 2025, you must kick off lead service line replacement projects
Watch the clip below where Greg provides the LCRR timeline overview.
Service line inventory requirements
Greg then provides more details about service line inventory requirements.
And provides a few ways to complete a service line inventory:
- Taking a brute-force approach, all hands on deck to tackle data collection
- Dedicating time and resource to get staff out on the field
- Hiring external resources to help take the load
- Using machine learning to assist in building out the inventory
>>YOU MIGHT ALSO LIKE: FAQS on Using Artificial Intelligence for Service Line Inventory Development
The inventory development workflow
Greg breaks down the elements involved in a service line inventory development workflow.
Desktop study: involves collecting data from various sources such as your GIS database, CMMS database, interviews with retired operators, and so on.
Rule-based assumptions: include common-knowledge best guesses; for example, certain pipe diameters don’t fit the typical size of lead pipes. Some utilities use customer self-reporting, a low cost method for collecting data. Customers are about 95% accurate when it comes to identifying copper; however, not as accurate with lead.
Recommended field verifications: using rule-based assumptions to make recommendations on where to conduct field verifications.
Perform field verification: starting the actual field verifications
Validate inspection: performing quality assurance/quality control (QA/QC) of the data collected thus far; this critical step ensures that you’re keeping bad data out of your machine learning model
>>YOU MIGHT ALSO LIKE: Developing a Living Inventory
What is machine learning as it relates to predicting lead
Machine learning uses data to learn and perform pattern recognition such as linear regression or logistic regression. For locating lead material, machine learning uses a clustering/classification approach. The model uses a training dataset to create a baseline of which to base its decision making to ultimately determine the probable presence of lead. Mark Zito, Trinnex’s product leader and senior solutions consultant, provides an overview of how this works.
When to introduce machine learning
Introduce machine learning once you have a baseline inventory set up. Create a feedback loop and continue performing field verifications to enhance machine learning model accuracy. You can advance your pipe prediction machine learning model up to three tiers.
Tier 1
A rule-based algorithm, simplistic method where you’re doing queries in a database and applying assumptions (such as using the size of the line and installation dates)
Tier 2
Automated machine learning where as field verifications come in, the model runs in the background producing a score (high, medium, low) of lead versus non-lead
Tier 3
Adds a data scientist layer to conduct exploratory data analysis and identify potentially adding data sets such as Zillow property data that might not be available; This extra step helps achieve a higher level of accuracy sooner in the process
Mark explains more about the three different machine learning tiers in this clip.
The typical development process for machine learning includes:
- Assessing data completeness and inferring any missing values
- Pre-processing data for optimal modeling
- Handling class imbalance and performing feature engineering
- Applying appropriate models to predict pipe material
- Testing predictions against real data to ensure model is reliable
- Continuously retrain model with new data and conduct “human in the loop” reviews
Why use machine learning for LCRR compliance? Key Takeaways
Machine learning offers many benefits for utilities to leverage as they work towards meeting LCRR compliance.
- Guides where to perform field investigations
- Utilities can save several thousand dollars by improving the hit rate of inspections (saving up 10% of total inspections) and avoid unnecessary digging
- Provides confidence in assumptions
- Machine learning helps to reduce the burden on staffing resources and the community by leveraging existing and newly collected data
- Inventory development can accelerate as the machine learning model becomes more accurate
- A machine learning model built into a living data management platform like leadCAST can produce reports to show LCRR compliance
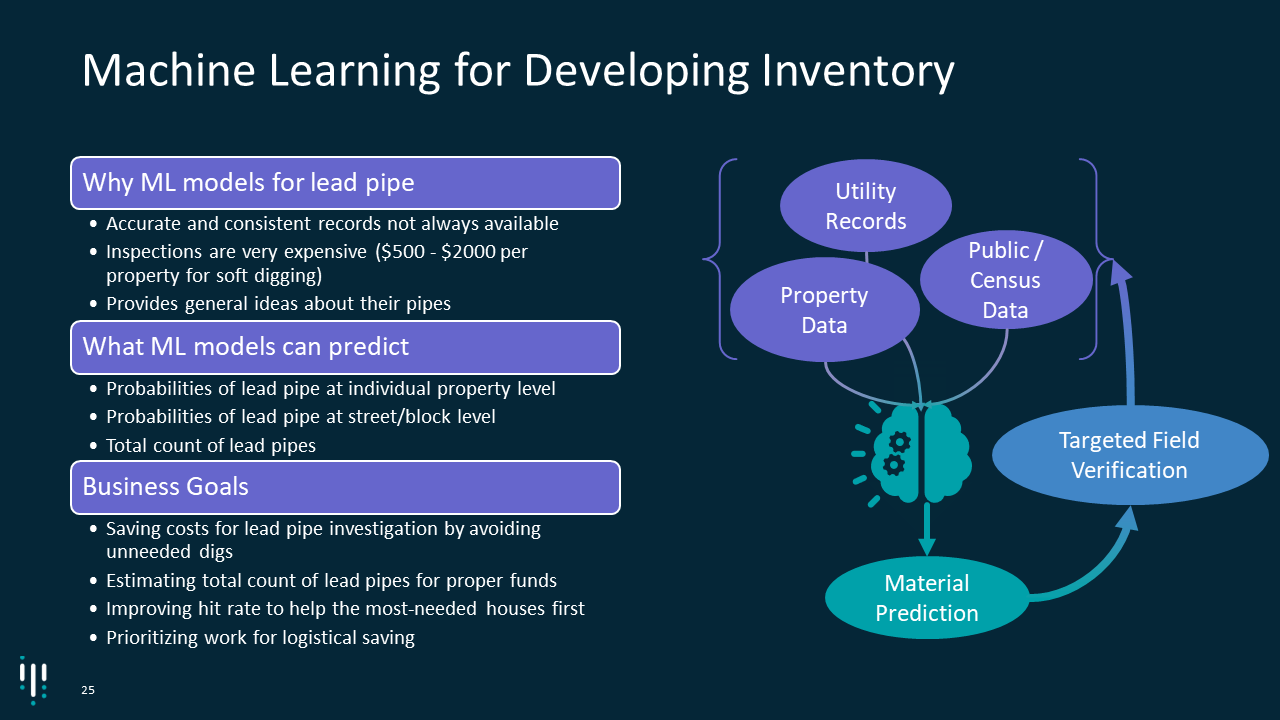
Choose your best machine learning path
Learn more about how machine learning works by listening to the full webinar recording. Ready to gain valuable insights by using machine learning available today through leadCAST? Schedule a 30-minute leadCAST consultation to learn which machine learning model works best for your current data situation.